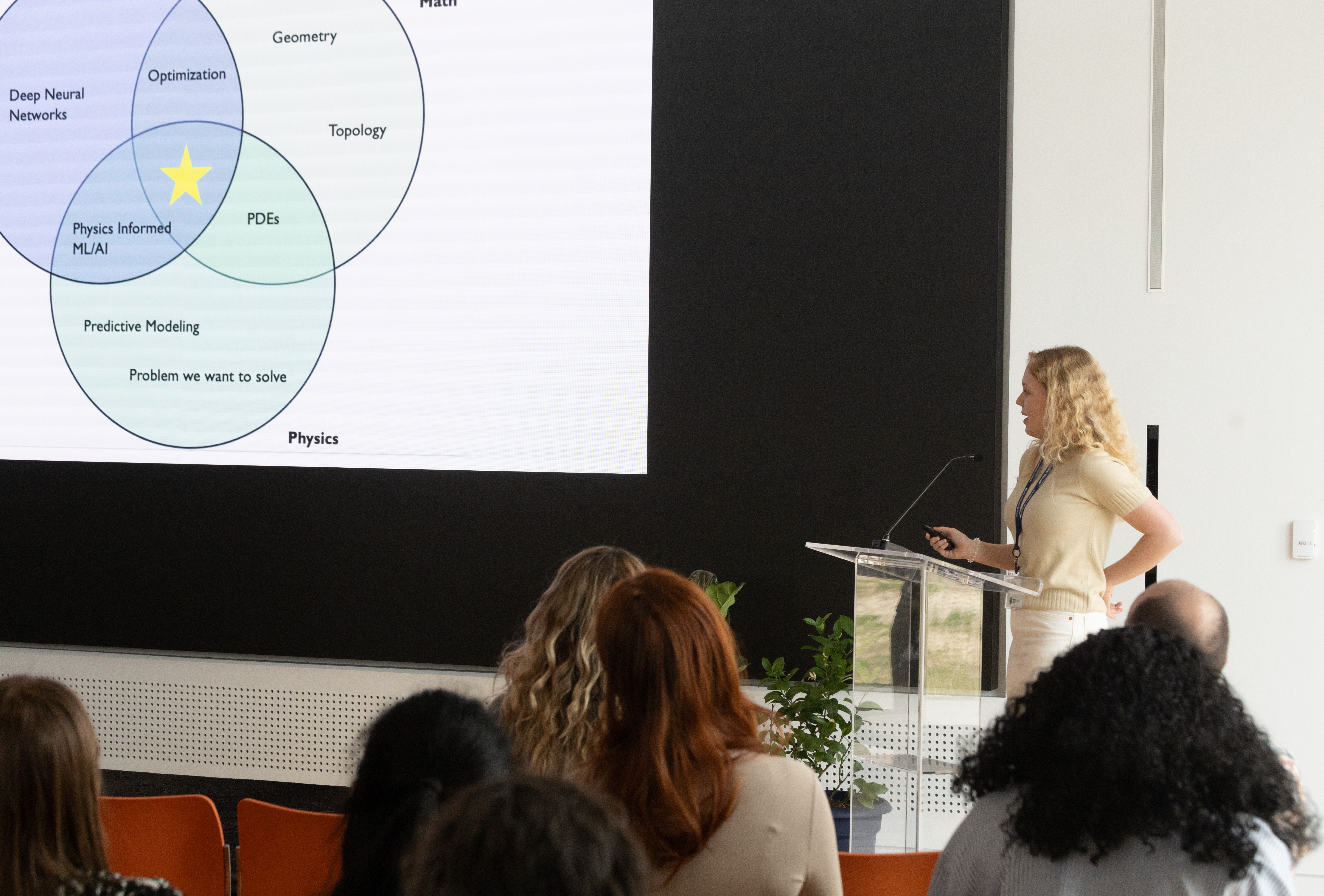
WiDS Lightning Talks Highlight the Innovative Research of Female Data Scientists
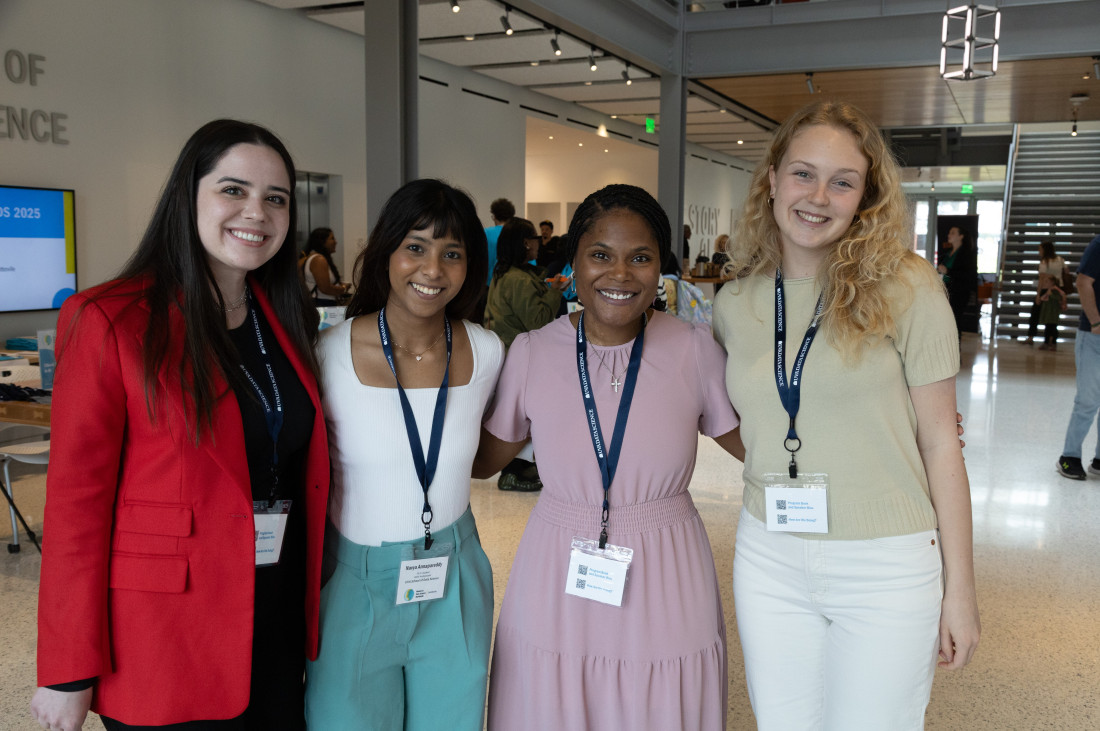
Women in Data Science Charlottesville 2025, held March 28 and for the first time at the new UVA School of Data Science building, featured speakers from a variety of research backgrounds, all aiming to share their insights and highlight outstanding women doing outstanding work.
One of the event's breakout sessions, "Research Lightning Talks," spotlighted four emerging data scientists and their captivating research. The session featured presentations by Ph.D. in Data Science students Zoë Gray, Kaleigh O'Hara, and Jade Preston and Ph.D. in Computer Science student Elizabeth Palmieri.
The students engaged in seven-minute presentations of their current research and projects of interest, with time set aside for audience questions. The goal of this session was not only to demonstrate the complexities and findings of their research but also to practice the ever-important soft skill of communication. The students presented data science concepts in a way that was easily digestible to non-technical audiences.
Gray began with her research, "Reduced Order Modeling of Dynamical Systems of Energetic Materials Using Physics-Aware Convolutional Neural Networks in a Latent Space (LatentPARC)." She discussed modeling energy materials using deep learning, achieving predictions in seconds compared to weeks.
Energy materials, Gray explained, are "materials which store energy which can be released very rapidly when an external force or chemical reaction occurs. These materials are used for pyrotechnics, explosives, and propellants." She examined the microstructure of such materials to evaluate the effectiveness of the model, aiming to quickly test different microstructures and materials to improve their precision and safety.
Gray concluded by proposing a lighter model called LatentPARC to reduce computational costs and training time while maintaining high prediction accuracy. She noted that she is currently diving into previous work that has been done in these areas, where physics knowledge is imbued into deep-learning architectures.
"With a little bit of knowledge from math such as geometry or topology as well as optimization and how partial differential equations work, we can try to optimize these architectures to make them more efficient and take up less computing power," Gray said.
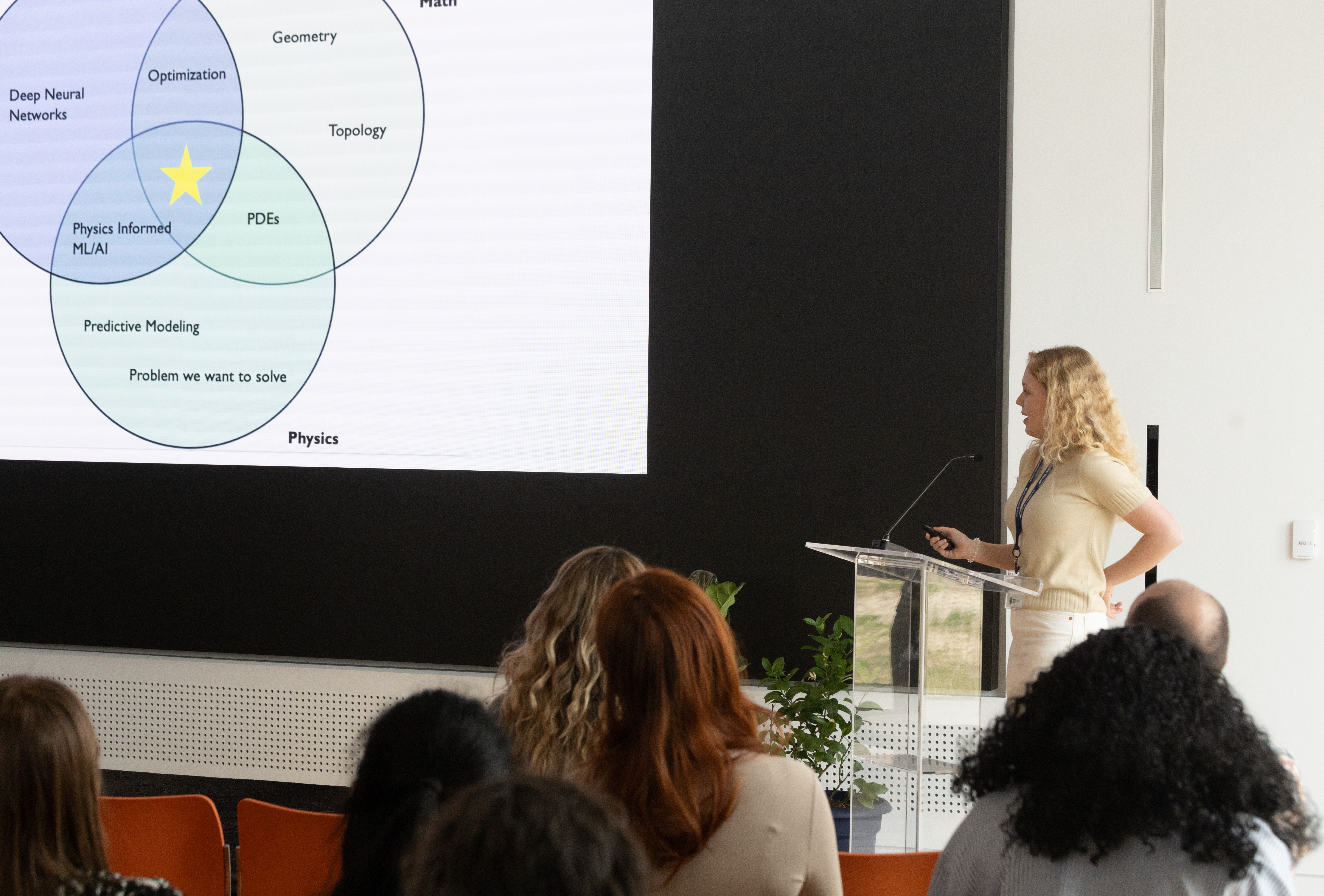
O'Hara followed with her research presentation titled, "Leveraging Machine Learning Techniques to Analyze Pediatric Cardiopulmonary Exercise Testing Data." O'Hara presented research on cardiopulmonary exercise testing (CPX) data, focusing on entropy differences between males and females across pubertal stages.
O'Hara began by detailing the CPX data, which is data collected while a participant runs on a treadmill about their breathing and heart rate, and also defining entropy. "Entropy tells us how uncertain things are. It explains the uncertainty in the data," O'Hara explained. "So, if data is more predictable, then there is less entropy."
For example, she noted that a system with low entropy would be sunrise time. We can all look at our phones and see to the minute what time the sun will rise, she said, depending on what city or neighborhood we're in. "There's low uncertainty if the sun will rise, therefore low entropy," O'Hara summarized. In contrast, a system with high entropy would be a coin flip. "If you were to flip a coin, you wouldn't know for certain if it would be on heads or tails prior to looking at it. So therefore, that is high entropy."
Her definition of entropy was accessible and appreciated by the crowd, with one audience member remarking: "I learned what entropy meant just from your presentation. That was a very good description."
O'Hara concluded that participants in early puberty tended to have higher entropy in their metrics during the test than their late-puberty counterparts, with a slightly higher increase in entropy for female participants than male participants. "One theorized reason for why early female participants have higher entropy is that as children approach adulthood, you're fine-tuning your physiology," O'Hara said.

Next, Palmieri presented "Contrastive Learning in Aspect-based Text Summarization." The goal of her research was to create concise, informative summaries guided by specific aspects.
She began with an example featuring Thomas Jefferson. After presenting a document of Jefferson's biography, Palmieri explained the problem of text summarization if the user is only interested in one aspect, like the founder's time at UVA, for example. "This is the task of aspect-based text summary," she said. "We have an input document, and we have the addition of an aspect which is guiding the model, showing the model what we're really interested in with the summary."
Palmieri noted how this kind of test is quite difficult for a model to perform, with the added aspect complication of limiting what information it can use while being able to make connections with other documents and information. "So, in order to have the model be able to successfully complete this task, we needed to contrive some sort of methodology to help the model be able to draw correlations between related information and then create space between congregated information. And we leverage this through contrastive learning," she said.
This methodology involves creating negative examples to train models, including using evaluation metrics like Chat GPT-4 critiques of the results. Overall, Palmieri explained, results showed that contrastive fine-tuning outperformed other methods. "In conclusion, this project is the first systemic analysis of [large language model] performance on aspect-based texturization utilizing improvement," she said.
Lastly, Preston, an active-duty operations research analyst in the U.S. Air Force, presented her research topic, "Advancement of Hyperspectral Image Unmixing and Analysis: An Application in Mineral Detection and Identification." She explained that this research on hyperspectral imaging for material identification was a part of her doctoral dissertation, which she recently successfully defended, and that it has applications in humanitarian aid, military operations, and disaster relief scenarios.
Preston began by establishing that hyperspectral imaging captures the unique spectral signature of materials, and that every material on Earth has a distinct signature. The resulting images contain pixels that have multiple materials, so the process of identifying materials and estimating their proportions in a pixel is called spectral unmixing.
"Spectral unmixing is commonly interpreted as a regression problem, and so for my contribution to the field of unmixing, I made a thorough comparison of regression-based approaches for unmixing," Preston explained. "Through that analysis, I developed a novel approach for unmixing the pixels that addresses a key misalignment of ordinary least squares within spectral unmixing."
Preston concluded by providing a novel taxonomy discussing the physical-chemical attributes of the materials that aid in their mixing. "I use that taxonomy to further identify the attributes of the material that created their successful detection," she said.
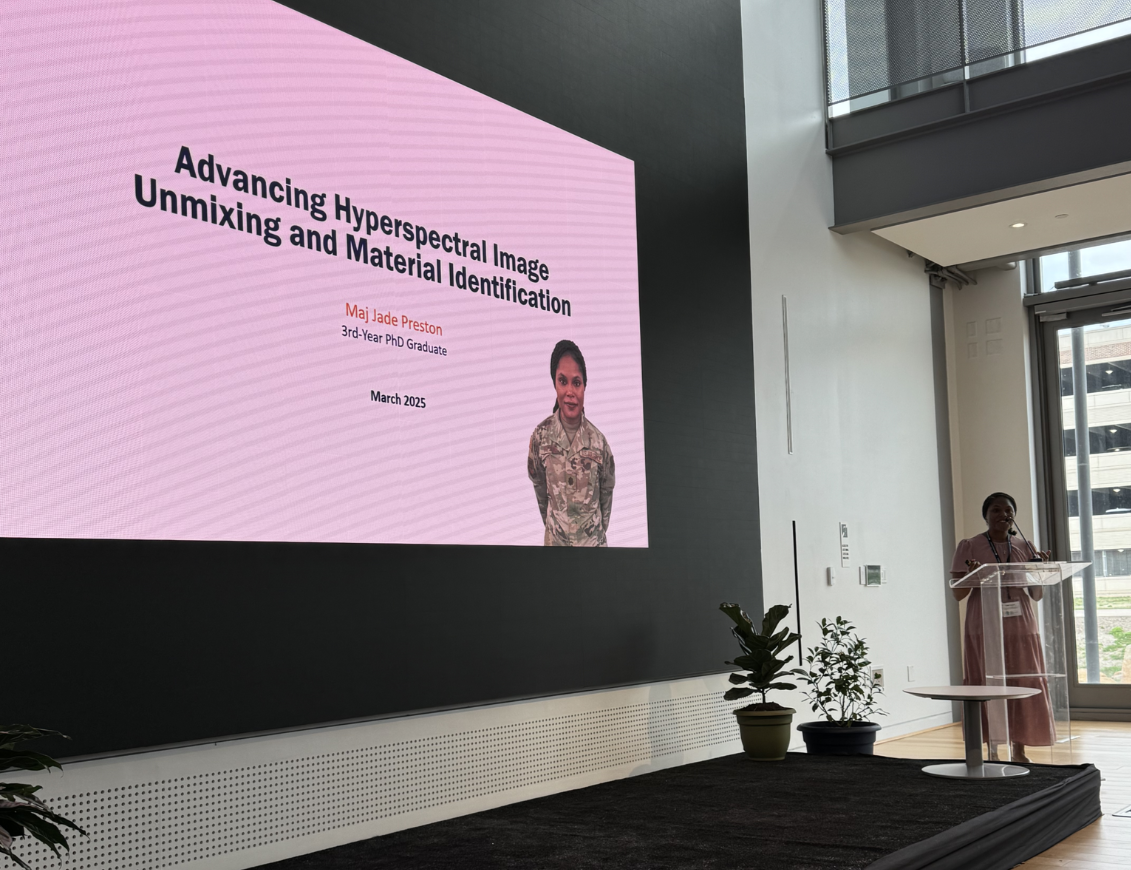