Self-driving cars, known as autonomous vehicles (AV), have been involved in 26 accidents since 2016, resulting in four fatalities. As more companies begin to invest in this technology, AV could be operating at scale on public roads in as little as two years and the safety of drivers, passengers and the public will become a major issue.
AV operates using sensory technology – data from sensors placed around the vehicle is rapidly input into statistical models that help the AV navigate roads. Although the technology is in many cases effective, even the most advanced sensor systems encounter difficult situations. In these cases the AV alerts the driver for manual intervention, although sometimes these notifications do not come early enough or they startle the driver and hinder their decision-making abilities.
UVA Data Science Institute Presidential Fellows Erfan Pakdamanian and Nauder Namaky aim to develop a system that optimally alerts drivers when the AV algorithm fails. They plan to develop a deep neural learning algorithm that considers external hazards in conjunction with drivers’ cognitive states.
Their algorithm will predict issues requiring manual intervention and will alert the driver accordingly, with different types of notifications (visual, auditory or a combination) for different hazardous situations. Pakdamanian and Namaky hope to develop a system that is the most efficient and safest way to transition from autonomous to manual driving while the vehicle is in motion.
The researchers will create this algorithm based on data they collect about how drivers operate vehicles. They will measure how drivers’ emotions and stress levels are impacted by different driving scenarios, such as in heavy traffic or during inclement weather. This will help Pakdamanian and Namaky to design an alert system that best shifts drivers into an engaged cognitive state without causing anxiety that may impair driving performance.
This research study will be the first attempt to evaluate how driver alert systems can be effective when they are designed to account for drivers’ cognitive states. This project is also unique in considering information about drivers’ emotional well-being in response to different feedback modalities.
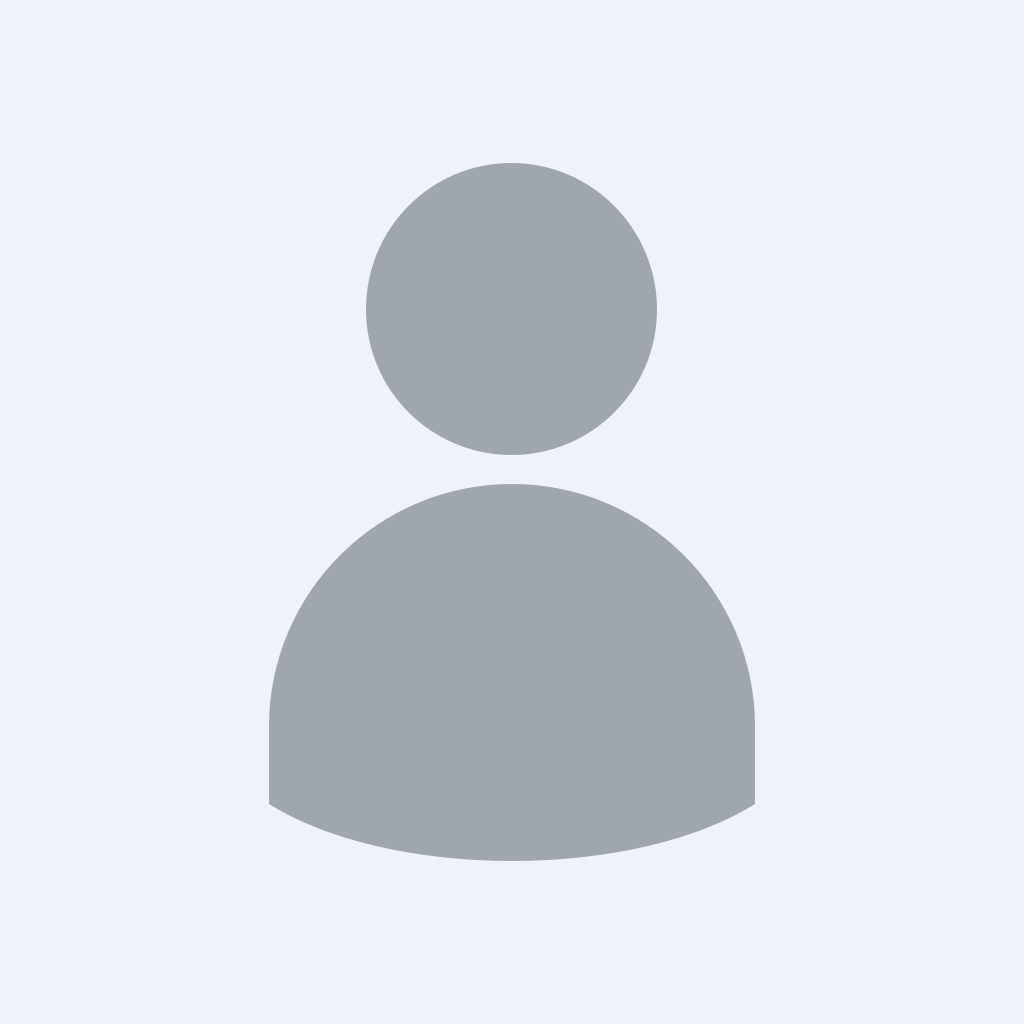
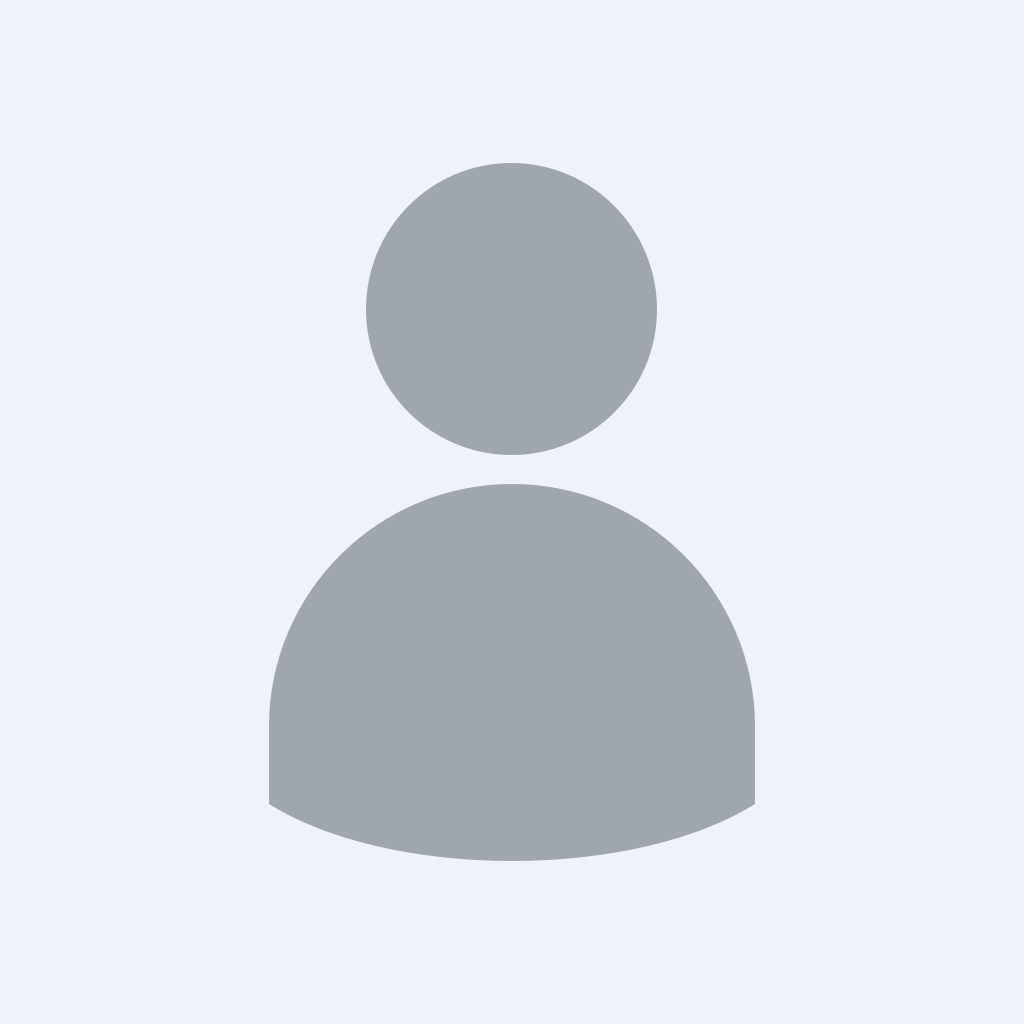