Biology has experienced an explosion of data characterizing the entire component list of cells and tissues. Hundreds of genome sequences, gene and protein expression arrays, genome-wide transcription factor binding data and genome-scale protein-protein interaction maps are available, necessitating the development of quantitative frameworks that integrate this data into predictive, computational models.
University of Virginia researchers Shayn Peirce-Cottler, Kevin Janes, Jason Papin and Martin Wu tackled this large-scale problem by building models to make predictions and experimentally validating these predictions to address a variety of health-care questions.
Shayn Peirce-Cottler focused on answering questions pertaining to the growth and regeneration of blood vessels, processes that are highly complex and span a wide range of spatial and temporal scales. Specifically, she was interested in understanding how blood vessels respond to environmental changes that are elicited by such diseases as diabetes, cancer and heart disease.
The model enabled her to explore the following questions:
- How do changes in blood flow to a tissue impact the regenerative capacity of vascular cells within that tissue?
- Which cell types invigorate a vascular growth response, and how do biochemical signals orchestrate this process?
- What is the role of stem cells?
- Why do vascular beds in different tissues behave differently from one another?
- What gives rise to person-specific variability in this context?
Kevin Janes addressed key questions in the regulation of intercellular processes. A major component of cellular regulation involves diffusible ligands, which bind to transmembrane receptors and thereby activate multiple signaling pathways inside the target cell. Measuring the function of these pathways, however, has tended to be time-consuming and limited in the number of samples that can be handled simultaneously.
Janes and his team approached the challenge of signaling-network measurements from an engineering process, actively using, refining and developing data-driven modeling approaches that can be immediately applied to multi-type biological data.
Jason Papin studied metabolism and transcriptional regulation in microbes and human cancer cells. The activity and control of metabolic processes are fundamental to:
- the growth of a microbial population in its environmental niche as well as in pathological settings, and
- the uncontrolled proliferation of a cancer cell, leading to tumor development.
Metabolic and transcriptional networks are highly interconnected, with thousands of genes associated with thousands of reactions interconverting thousands of metabolites. Papin’s work developed computational methods to integrate these multi-type data to generate predictive models of cellular function and high-throughput methodologies to validate these predictions.
Martin Wu focused on how microbes living on human bodies (also known as human microbiota) affect health and disease. Microbes encompass thousands of different bacterial species and form dynamic and complex interaction networks that aid in the metabolism of nutrients, compete with pathogenic bacteria and modulate the development of the immune system. He developed methods to synthesize meaningful ecological units of bacterial species from this massive data collection and used novel statistical approaches, such as the maximum information coefficient to mine millions of sequence reads to detect interactions between bacterial species.
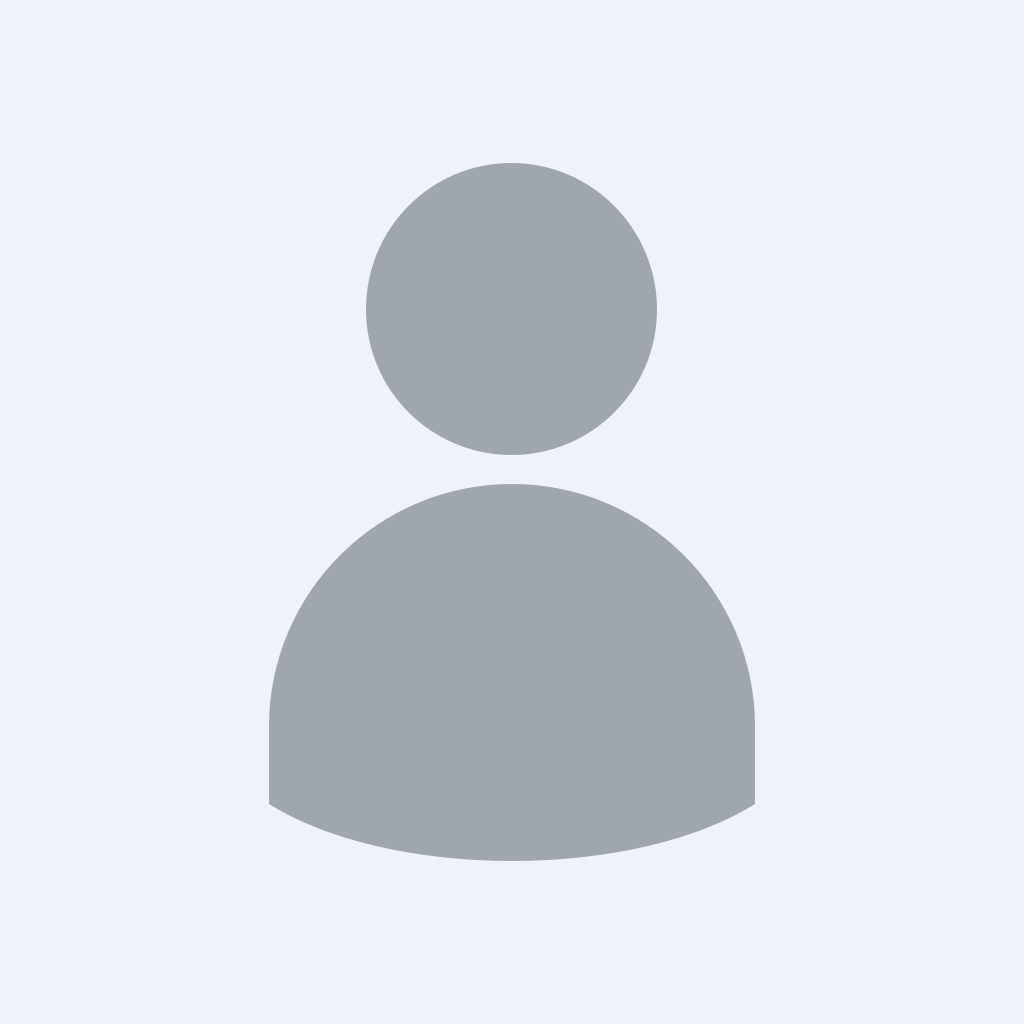
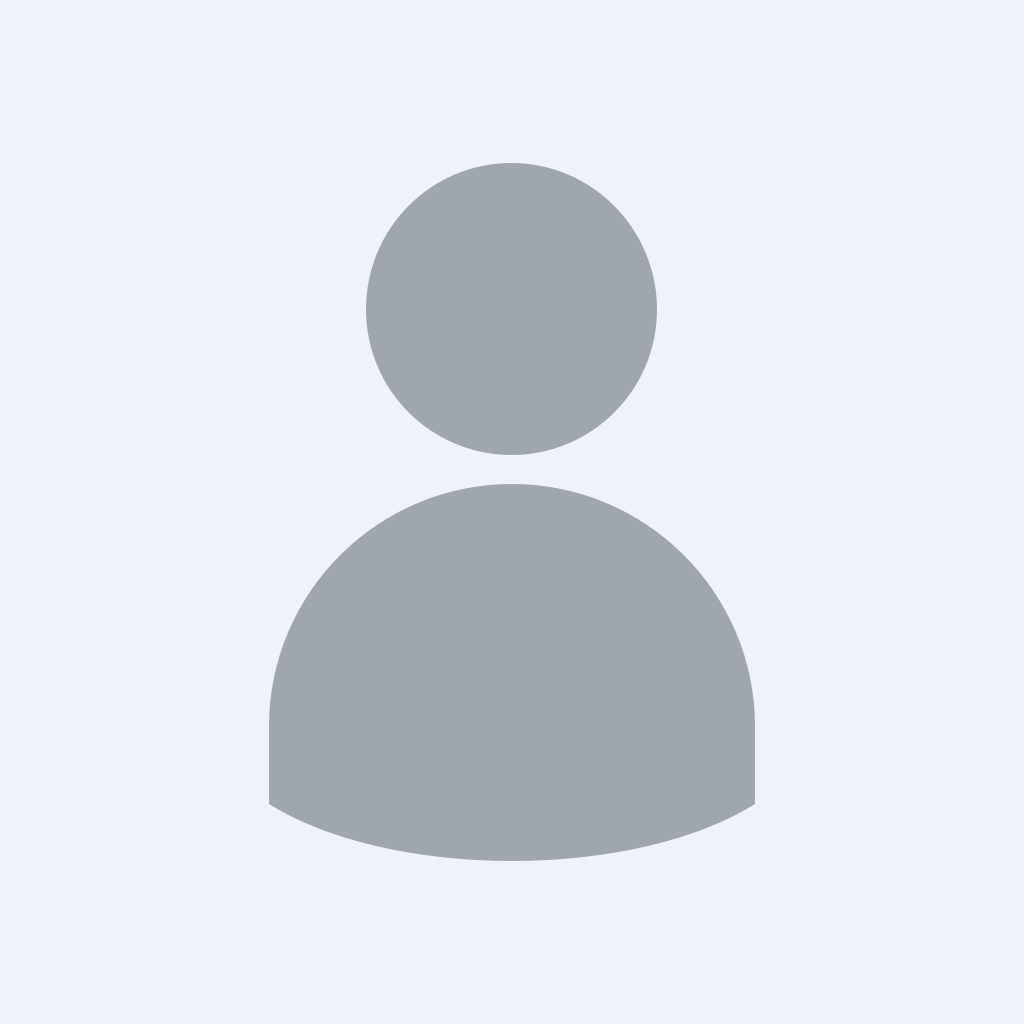
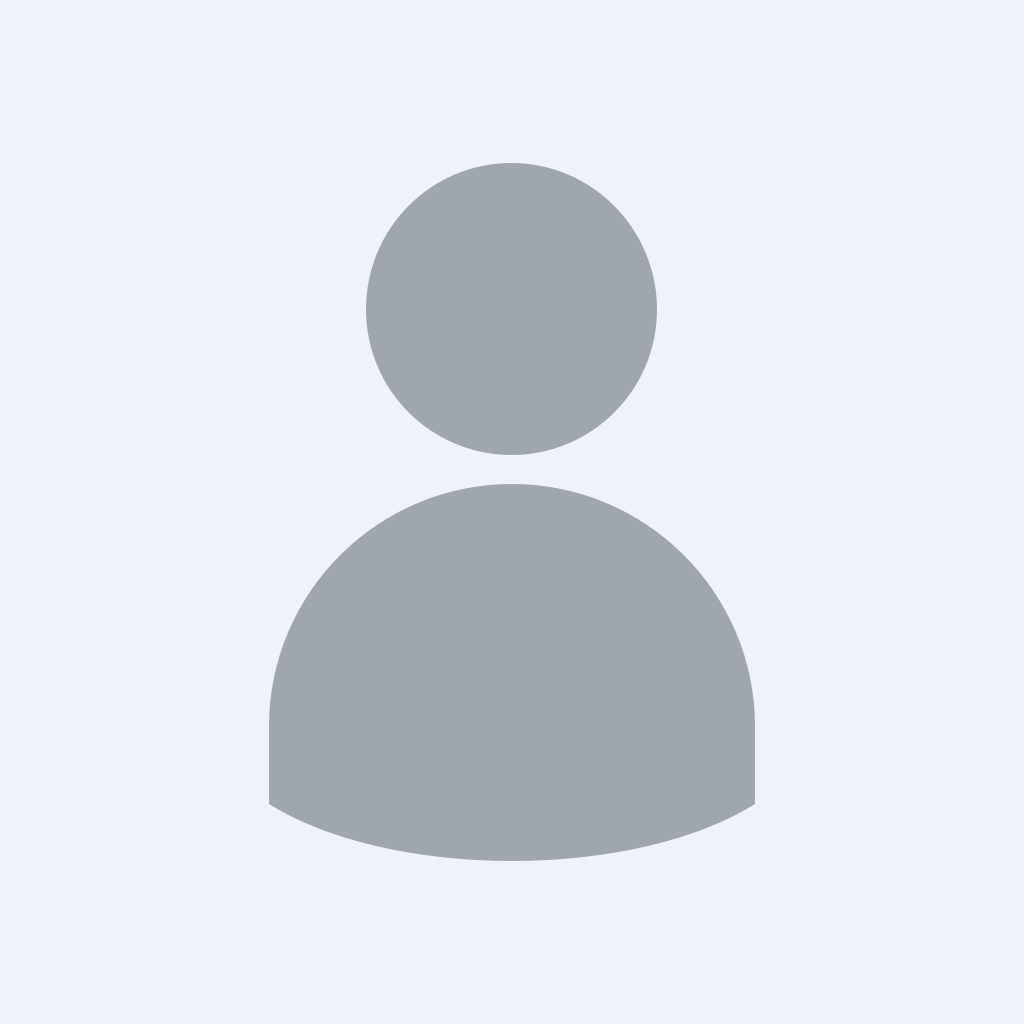
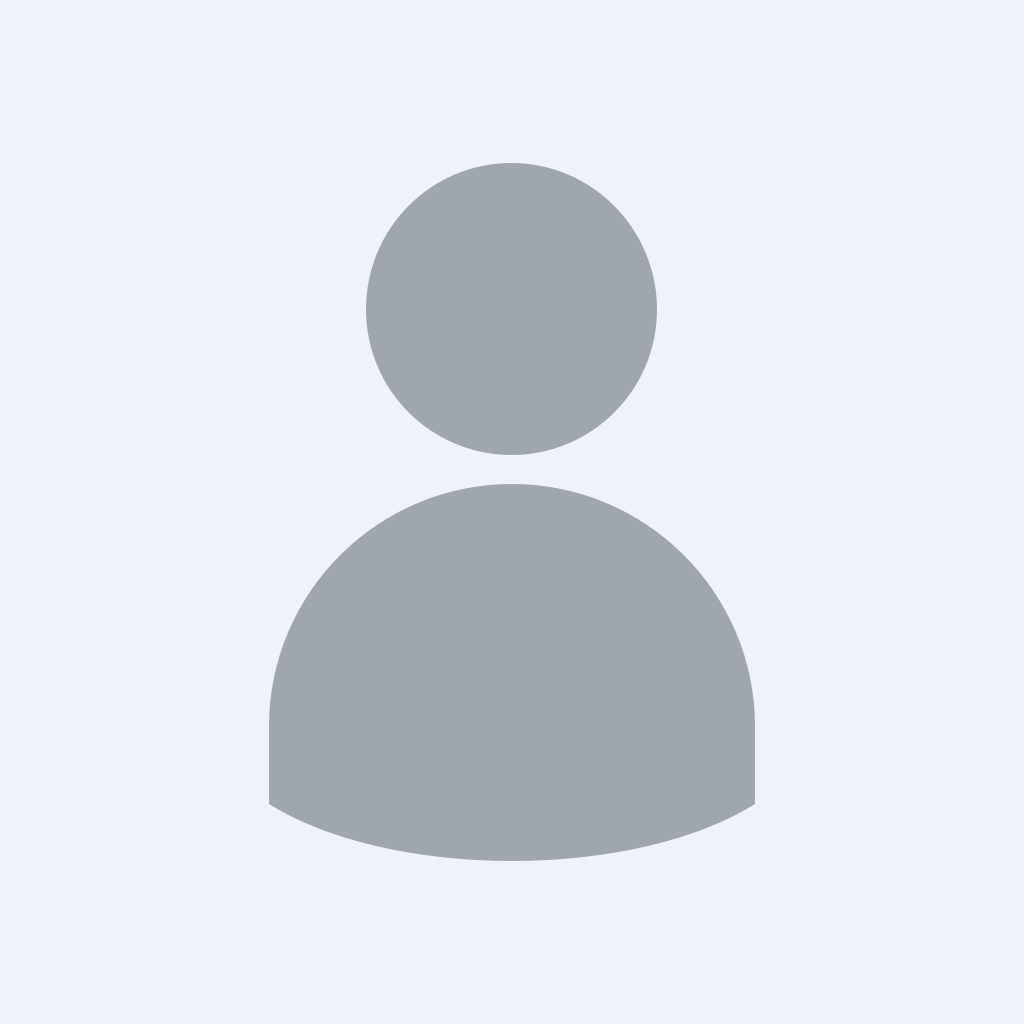