What Is the Difference Between Data Science and Data Analytics?
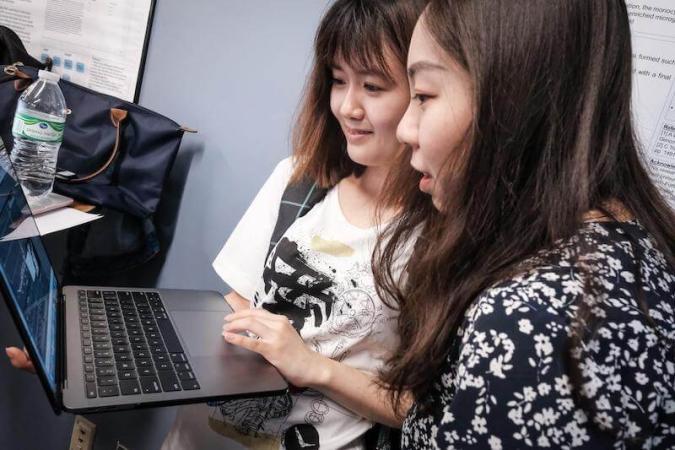
Data analytics and data science are both popular fields right now, and there is a high demand for qualified employees who demonstrate skills in each of them. In fact, a recent study suggests that the combined demand for data professionals will be over 2.7 million by 2020, up from 2.3 million in 2015. While much of the work in data science vs data analytics overlap-they both work with data and generate insights from large data sets-there are important distinctions between the positions. In general, data scientists generate questions to ask while a data analyst responds to questions from the business side. Additionally, data scientists generally have a stronger technical foundation, although technical skills are useful for data analysts as well. Data analytics may have more business experience, which benefits them when working cross-functionally.
Key elements of data analytics
A data analyst manipulates and uses raw data to answer questions for the business and product teams. A data analyst will work more closely with business teams, so one with an interest in driving strategic business decisions might be more interested in data analytics. A data analyst will typically take a question from the business side or a problem presented by someone else and gather the data to assess the situation. Then, they’ll turn this data into an actionable insight that can be understood by a non-technical stakeholder. Data analysts also generally spend more time on data visualization and presenting data than data scientists do, but data scientists still work extensively with visualizations. Data analysts hunt for trends in the data, perform advanced analytics strategies and they utilize techniques that help them generate an insight and data analysis.
Key elements of data science
A data scientist also uses data to generate insights for a company, but they might use more sophisticated models and combine data from a variety of sources. Learning how to take those insights and making them actionable over time is a valuable contribution to any company. Additionally, their insights might not answer immediate business questions-they may instead provide a longer-term forecast for the company. A data scientist usually spends most of the day programming, generating statistical models, and cleaning data to generate insights from it. They often work closely with engineers to understand exactly where the data is coming from so that they can more effectively normalize it. Like data analysts, data scientists are responsible for anticipating needs from the business side of the organization and finding the data to help them, but when compared to data analysts, their role is less business-oriented. They analyze and mine large data sets, and they use tools such as R and Python to generate insights from the data. Additionally, they may work with artificial intelligence (AI) and Machine Learning models to go deeper into massive data sets.
Connections between data science and data analytics
Regardless of the differences between data science vs data analytics, many companies are looking for both data analysts and data scientists to fill critical “big data professional” roles within a company. In the past five years, a booming number of industries-such as education, entertainment, retail, healthcare, non-profits, and government have begun looking for data professionals, so there is no single type of industry that is seeking out data scientists or data analysts. Industries in which only a data analyst would be desirable are those that store smaller amounts of data. A data scientist will typically explore data from multiple sources and standardize it, while a data analyst is more likely to use data from just one source. As a result, a company that only has one source of data, such as a small retailer, might decide to hire a data analyst instead of a data scientist, particularly because data analyst salaries are lower.
Additionally, because data scientists are more likely to have a Ph.D. in statistics or mining massive data sets, a company with smaller data sets might seek out a data analyst who would be happier working with smaller sets of data. A company may hire only a data scientist, rather than a data analyst if much of their analytics work is being handled by existing teams and the company seeks a professional who can dig into exploratory data. Many smaller companies seek data analysts before data scientists, and many data scientists searching for jobs are actually data analysts with programming skills. For a company that doesn’t need a programmer, a data analyst can be the right hiring choice. A company would prefer a data scientist if they’re looking for someone to mine data for machine learning and intelligence insights, rather than business analytics. Both roles are extremely valuable, and whether or not a company is hiring one or the other depends on their overall data needs.
Careers
Skills and abilities
Data analysts and data scientists require similar skills, but data scientists are more technical while data analysts are more business-oriented. Mining data is an important skill set for both. The most commonly-cited skills in data analytics job descriptions are SQL, Excel, and R while low-level programming languages like Python and Java are cited more often in data scientist job descriptions. Additionally, data analyst job descriptions put a stronger emphasis on communication skills because of the amount of time data analysts will interface with the business side.
Education and experience
Over one-third of job postings for data scientists and data analytics require a master’s degree, and this can be useful for someone looking to break into either field. Most master’s programs in both data analytics and data science list a STEM background as desirable. However, data analytics master’s programs can be more lenient in their requirements and skills. A candidate with a background in the humanities or social sciences who has some coding and statistics skills would be qualified for many data analytics master’s programs. Data science master’s programs typically prefer candidates who have an undergraduate degree in math, statistics, or engineering. For candidates interested in data science who haven’t worked in technical fields before and don’t have the tools necessary, a program like University of Virginia’s Master of Science in Data Science is an excellent way to gain the technical foundation needed to excel in the field.
A variety of undergraduate majors are possible for both data scientists and data analysts. The best majors for both data scientists and data analysts are those in the STEM fields such as math, engineering, statistics, and computer science. Economics can also be a relevant major for becoming a data scientist and a data analyst, particularly if you have worked on research projects involving high volumes of data.
Internships
Internships are a helpful way for a college student to break into data science and data analytics before graduation and gain the right tools. Many large tech companies like Google and Facebook offer formal internship programs that students can apply to online. Smaller companies are also often open to interns but might not have a job listed formally, so students should try to reach out to people who work at the company. However, internships at a larger company typically provide more training and structure projects for the interns to understand what data science and data analytics at that company is like. For a data analyst, working with business teams is critical, so finding an internship that allows the intern to work cross-functionally with other teams and focus on deep analysis of the projects can help them break into the field successfully. Additionally, many data science internships are seeking candidates with a research background, so studying a master’s or a Ph.D. can help a data science candidate gain an internship.
Bootcamps
A number of bootcamps are emerging to train data scientists and analysts in a matter of months for a fraction of the cost of a master’s program. The main caveat with some of these programs is that candidates have to already have a strong technical and analysis background going into the bootcamp. In fact, many specifically want people with Ph.D.’s in technical fields. However, if you’re currently a data analyst looking to transition into being a data scientist, a bootcamp could be a more efficient way of doing that. Bootcamps are less common for data analysts because companies typically want data analysts with strong business and communication skills which can be more readily developed by working rather than at a bootcamp. For someone interested in pursuing a career in the field, a master’s program offers a more comprehensive introduction.
Background in business
Another educational option for data analysts is business school. As data continues to be a driving force in decision-making, a number of MBA programs are starting to offer analytics tracks. For someone interested in pursuing data analytics and then transitioning into an executive role overseeing the data, an MBA might be the right educational choice to gain the important tools and skill set needed to continue growing in your career and learning new skill sets.
Differences between data science and data analytics
Comparing data science vs data analytics results in a number of differences as well. In general, the data scientist role is more technical, while the data analyst role carries more business acumen, although this varies based on the company. At many companies, data analysts are a support role for business teams, and a data analyst will be assigned a particular business stakeholder to work with. The ability of a data analyst to use data to answer questions that drive product and business changes is second to none, and data analysts often find themselves overloaded with requests to help. Data scientists, on the other hand, generally come up with questions to ask. Instead of letting business teams drive their analysis, they look at the data directly to figure out what the most relevant questions might be. They look for patterns in the data. They also use machine learning and Artificial Intelligence to find these patterns in the data that would not be noticeable via traditional statistics or visualization techniques. Both of these are critical tasks, but they take different approaches to optimize the use of data.
Aside from the overall job functionality of asking questions vs. answering questions, the day-to-day roles of data scientists and data analysts differ. Data scientists spend time modeling the data and coming up with new processes for looking at data. Data analysts often deep-dive into the nitty-gritty of databases and spreadsheets. Both data analysts and data scientists spend time cleaning data, so technical skills are critical for both, but a data analyst is more likely to work with Excel or a visualization tool like Tableau, while data scientists are more likely to work programmatically. Data scientists must be able to query databases as well, and they’re more likely to also be setting up the databases if they have the appropriate engineering skills. Ultimately, the objectives are similar in that both seek to help a business drive success by making use of its data. A data scientist may focus more on longer-term success while a data analyst may be responding to day-to-day requests from business stakeholders.
The roles of data scientists and data analysts vary, and both can find themselves in a variety of positions. For example, a technical data scientist may also be qualified to take on data engineering roles. Data engineers are software engineers who work closely with data. They have strong software engineering skills that allow them to analyze the data. They use computer science methods to handle large amounts of data. A data engineer focuses on cleaning the data and building databases. Although data scientists do this as well, it’s a larger part of the job for data software engineers. Therefore, a data scientist with strong programming skills could have a role more similar to that of a data engineer.
A data analyst might take on roles more similar to that of a business analyst. A business analyst helps an organization improve efficiency and products by generating insights about the business, but these might not always be data-related. For instance, a business analyst may recognize an organizational inefficiency by interviewing members of a particular team. Because data analysts tend to have a stronger business background, they are more likely to take on this role if their company needs business analysts.
Demand for data professionals
Because of the increased ability to store and manipulate large quantities of data, many companies are looking for data professionals. The demand for data analysts and data scientists is lofty For data analysts, the demand is expected to increase by 19 percent from 2018 to 2020, with salaries for a senior data analyst beginning at $84,000/year and continuing to rise with demand. This demand for data scientists is expected to increase by 29 percent by 2022, and salaries start at around $106,000/year nationally. Both of these positions are experiencing an increasing shortage of qualified candidates, and the demand suggests that these are promising fields to enter.
Choosing between data science and data analytics
Data science and analytics are both in-demand fields right now, and for a candidate interested in working with data and learning how to gain that skill set, either would be an excellent career choice. Data analysis is relevant across so many job opportunities. The UVA MSDS prepares students for careers in a number of fields, including data science and data analytics. Because technical skills are becoming increasingly important, training to be a data scientist also prepares a student for a career in data analytics. Learning these skill sets opens many options for students that take this program. Additionally, the program offers advanced courses in AI and machine learning to prepare students for more sophisticated data science roles. The UVA MSDS program offers an extensive foundation in data science with coursework like Data Mining and Introduction to Computer Science, as well as an opportunity to work on a capstone project and gain the real-world experience necessary using all the tools you need to excel in any industry. Learn more about our programs today.
Now more than ever, data is shaping the future. Are you ready to contribute your curiosity, imagination, and energy to the world of data science?