Five Reasons Why Organic Data Is Healthy For A Data Science Model
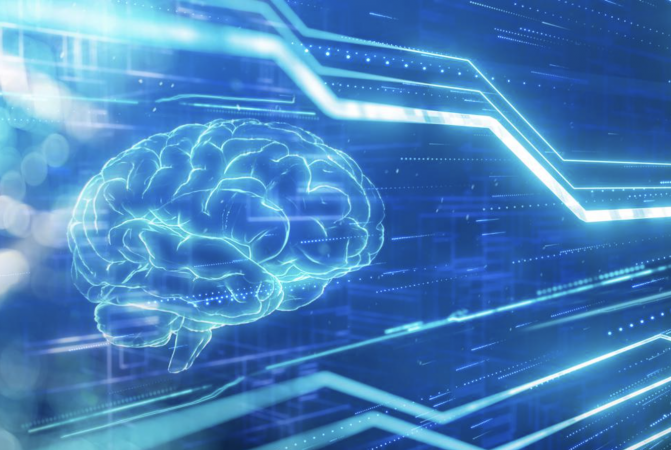
Sree Mallikarjun is a Chief Data Scientist at Reorg, a global provider of credit intelligence, data and analytics, and Adjunct Faculty at UVA’s School of Data Science.
Forbes, by Sree Mallikarjun
Published Sep. 15, 2021
Five Reasons Why Organic Data Is Healthy For A Data Science Model
Text data is one of the largest forms of unstructured data and is ever-growing. At Reorg, I work with large amounts of financial text data every day. One challenge of working with text data is that you need a large training data set to build robust models. You also need good, organic training data, which will be described in further detail in this article.
Machine learning (ML) models are only as good as the data used to train them. Over the years, I have collected training data to train several supervised ML models from databases where the data was labeled as part of some business process — or new training data from subject matter experts (SMEs), project managers and product managers. It is important to put in effort and time to ensure your training data is organic, meaning it is rich, robust and reliable.