Doctoral Students Present Summer Research Topics
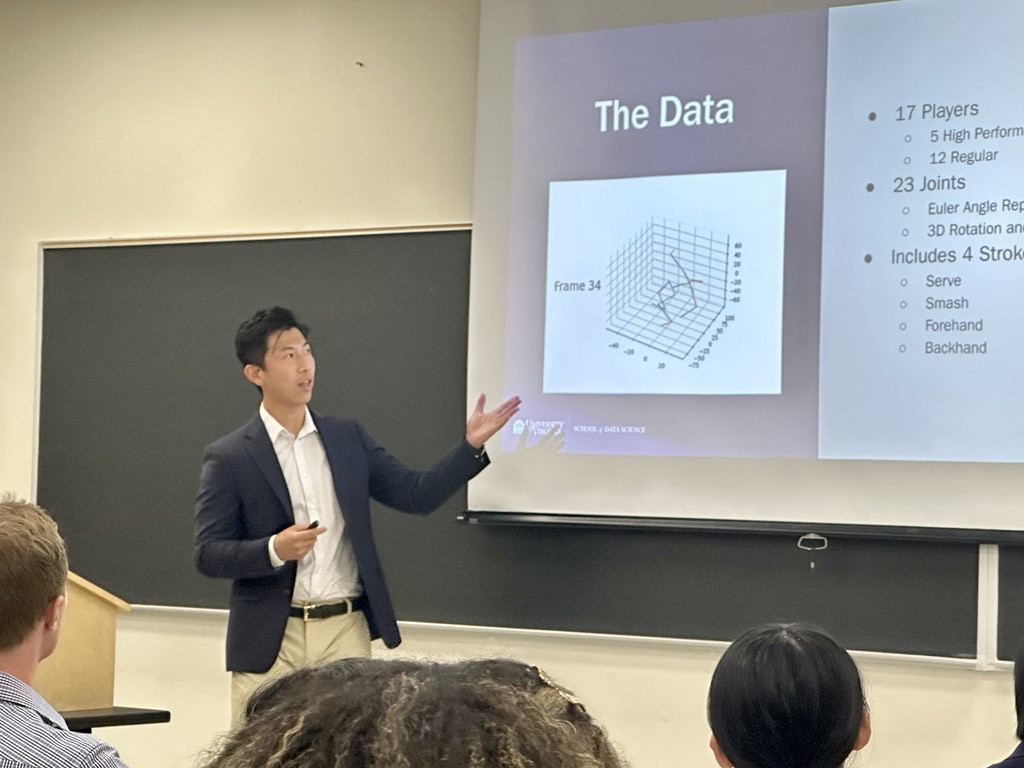
Second-year doctoral students at the University of Virginia School of Data Science presented their summer research projects as part of Ph.D. orientation day, welcoming thirteen new students. Program Director Tom Stewart kicked off the presentations to a well-attended audience of current faculty sponsors and new faculty members who were attending their own orientation simultaneously. The lightening research talks showcased the breadth of topics and introduced faculty sponsors. Twelve students presented, with research ranging from predicting food insecurity in Africa to optimizing smoothness and accuracy of tennis players.
Order of Presentations:
Meesun Yang
Supervising Faculty: Bill Basener
Abstract: In hyperspectral imaging, remote sensors are used to collect spectral data from images to identify various substances and /or processes in those images. This presentation will discuss some of the basics behind hyperspectral imaging, how it is used, and the significance of data science in this area.
Jason Wang
Supervising Faculty: Stephen Baek
Abstract: We present a novel approach to constructing a motion manifold tailored for tennis players, enabling comprehensive stroke and serve analysis. Leveraging an autoencoder architecture, our method attempts to preserve intricate local geometries encompassing distances and angles within the manifold. This manifold serves as a foundation for diverse downstream applications, including motion generation, action synchronization, and injury mitigation within the realm of tennis performance.
Gia Smith
Supervising Faculty: Jeffrey Blume
Abstract: ROC curves are used to measure the predictive accuracy of machine learning algorithms and statistical models. ROC curves show the sensitivity/specificity tradeoff of the algorithm or model, and the area under the curve is used as a summary measure of predictive accuracy. ROC models are complex because the ROC curve is invariant to monotonic transformations of the outcome, and the fitting algorithm must accommodate that flexibility. Here we examined the effectiveness of fitting algorithms for ROC models, namely Maximum Likelihood Estimation and different variations of Gradient Descent.
Jade Preston
Supervising Faculty: Bill Basener
Abstract: Food insecurity in Africa is one of the major global-scale humanitarian disasters. The United Nations (UN) World Health Organization estimates that 37 million people in the horn of Africa are in acute hunger and describe the current situation as one of the worst hunger crises in the last 70 years. In this talk we present a machine learning prediction using Neural Networks, tree models, and Bayesian model averaging to predict the change in food insecurity for each country in Africa along with proposed locations for new transshipment nodes based on the current food supply chain.
Karolina Naranjo-Velasco
Supervising Faculty: Rafael Alvarado
Abstract: The presentation explores the integration of natural language processing (NLP) within the legal domain. It showcases its utility in facilitating tasks such as information extraction, prediction, and other core tasks. Furthermore, it introduces the 'law as data' methodology, a transformative approach that converts legal texts into quantitative data, which can be processed by machine learning methods to reveal new insights. The presentation concludes by discussing the emerging trends and challenges in the field of computational legal studies.
Luz Melo
Supervising Faculty: Thomas Stewart
Abstract: In this study we investigate the effect of Ivermectin, Fluticasone, Fluvoxamine on symptom-specific recovery among patients with mild to moderate COVID-19. By focusing on individual symptoms, this re-analysis of the ACTIV-6 platform trial data seeks to provide insights into the efficacy of repurposed medications at alleviating specific manifestations of the disease.
Kevin Lin
Supervising Faculty: Don Brown
Abstract: Eosinophilic Esophagitis (EoE) is an allergic condition increasing in prevalence. To diagnose EoE, pathologists must find 15 or more eosinophils within a single high-power field (400X magnification). Determining whether or not a patient has EoE can be an arduous process and any medical imaging approaches used to assist diagnosis must consider both efficiency and precision. We propose an improvement of Adorno et al's approach for quantifying eosinphils using deep image segmentation. Our new approach leverages Monte Carlo Dropout, a common approach in deep learning to reduce overfitting, to provide uncertainty quantification on current deep learning models. The uncertainty can be visualized in an output image to evaluate model performance, provide insight to how deep learning algorithms function, and assist pathologists in identifying eosinophils.
Beau LeBlond
Supervising Faculty: Alex Gates
Abstract: Bibliometrics has become the gold standard of examining the impact of science, but many current methods only examine the impact of science within science. In this study, we examine the impact science has on policy and vice versa. We study the environment around general data protection regulation and thereby set up a methodology for other scientific disciplines to assess how their literature is received in the context of policy.
Zachary Jacokes
Supervising Faculty: John Darrell Van Horn
Abstract: Neuroimaging data presents unique challenges both in terms of scale as well as variability. My talk outlines the methods our lab has implemented to process, harmonize, and analyze these data when the variability is absurdly high as a result of having four different data collection sites.
Bryan Christ
Supervising Faculty: Jonathan Kropko
Abstract: Standardized tests play an important role in American mathematics education; yet, due to limited test banks, there is a severe lack of available questions for students to practice for these tests. Additionally, existing practice tests are not aligned with activating student interest, which is associated with increased test performance (Bernacki & Walkington, 2018; Walkington, 2013). To address these problems, we seek to create a Math Test Question Generator, a Large Language Model (LLM) that can create a limitless bank of math test questions aligned with student interests.
Zachary Blanks
Supervising Faculty: Don Brown
Abstract: Sample entropy is commonly used to quantify the complexity of time series signals, but it is sensitive to poor parameter settings, especially for short signals. We introduce a novel Bayesian optimization framework that systematically selects optimal values for the provided signal set. Through empirical analysis, we demonstrate that our method outperforms existing techniques in detecting physiological entropy differences among clinical study participants.
Navya Annapareddy
Supervising Faculty: Stephen Baek
Abstract: This research centers around differential geometry and representing human motion meaningfully through the use of human pose estimation and manifold learning. She applies the motion manifold theory to help detect movement disorders like cerebral palsy in clinical settings and make their diagnosis more interpretable.